Day 1 :
Keynote Forum
Ken Williams
KenAnCo Biostatistics and University of Texas Health Science Center at San Antonio
USA
Keynote: Individualized medicine: some of the ways biostatisticians can help
Time : 09:30-10:00
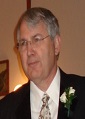
Biography:
Ken Williams received a BS in applied math from Georgia Tech in 1971 and an MS in Operations Research from the Air Force Institute of Technology in 1980. He served in the US Air Force for 22 years in Computer Systems and Scientific Analysis. He also served 10 years as a Biostatistician at the University of Texas Health Science Center at San Antonio where he remains an Adjunct Faculty Member. He has been a Freelance Biostatistician with KenAnCo Biostatistics since 2007. Designated a Professional Statistician (PStat) in the inaugural 2011 litter, he has published more than 100 papers in peer-reviewed journals.
Abstract:
There is a growing trend toward more individualized treatment in clinical practice. This talk will focus on three advanced research technologies which biostatisticians can apply to support physicians’ individual patient treatment decisions based on each patient’s attributes such as age, sex, questionnaire responses, blood pressure, lab values, and genotypes: (1) Models which quantify the benefit each patient can be expected to receive from a particular therapy. For example models quantifying the expected benefit from lipid-lowering statin treatment can identify younger lower-risk individuals who would meaningfully benefit as much as higher-risk older individuals and thus enable earlier initiation of therapy before the “horse is out of the barn”. (2) Comparative effectiveness research using benefit models to identify the best of multiple therapeutic options for each patient based on his or her particular attributes. An example of this is research aimed at distinguishing between patients would receive a net benefit from statin treatment despite increased risk of diabetes and those expected to have a greater net benefit from some alternative therapy. (3) Cautious use of meta-analysis across multiple studies using an individual-level pooled dataset to estimate parameters estimates based on identically designed models fit within each study. A good example of this approach is the Cholesterol Treatment Trialists collaboration which used meta-analysis combining 22 different clinical trials to find that within trial relative risk reduction from statin treatment was lower for patients with higher baseline risk than for lower risk patients. Their findings were applied in the benefit model mentioned in (1) above. I’ll also give a bad example during the talk.
Keynote Forum
Abbas F Jawad
University of Pennsylvania
Children’s Hospital of Philadelphia
USA
Keynote: Cluster randomization trials in schools setting: design and analysis
Time : 10:00-10:30
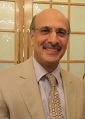
Biography:
Abbas F. Jawad has earned his MSc (1986) and PhD (1993) from the Graduate School of Public Health, University of Pittsburgh, USA. He is an Associate Professor of Biostatistics in Pediatrics at the University of Pennsylvania Perelman School of Medicine, Department of Pediatrics at the Children’s Hospital of Philadelphia.. He has published more than 100 papers in reputed journals and has been providing biostatistical support for medical pediatric research for more than 20 years.
Abstract:
Cluster randomization trials (CRT) are commonly used in the evaluation of non-theraputic interventions such improvement of educaiton programs, health educations and enovation in behavioral and enviromental improvement in schools and communities. Cluster as a unit of randomization varies in in sizes, It could be a housholds or families, entire communities, religious institution, hospitals units, classrooms. Unlike individually randomized trials, CRT can measure the interventions efect on a targetted group of individuals. However, it prones to be less efficient with potentially weaker statistical power, hence requires more clusters. During the past two decade, CRT designs and methodology has been intensely improved, although scattered, it cover a wide range of applications. Fisher’s thoery of experiemtal desing assumed that the randomization unit is the same unit of analysis. The uniqness of CRT is that the randomization unit is the clusters, and the analysis target members of the clusters. In school setting,measurements obtianed from students within a school are expected to be more correlated than measurments obtianed from students in a different schools, similarly, for classes within schools. Such correlation should be accounted for during the statistical analysis stage. Another source of chalanges are related to the collaboration of the gatekeepers and stakeholdre in these schools, reliability of tools used, consistency among therapists, and teachers. Also, seasonal effects as well as uncontroled school‘s changes related to personnels and budget cutting. CTR require approval of the athical committees desgniated witin schools, which requires better understanding of study design since gatekeepers can’t consent on behalf of students.
Keynote Forum
Altaf H Khan
King Abdullah International Medical Research Center
National Guard Health Affairs
Saudi Arabia
Keynote: Some applications of Fourier /wavelets transform in Statistical Sciences
Time : 09:50 - 10:15
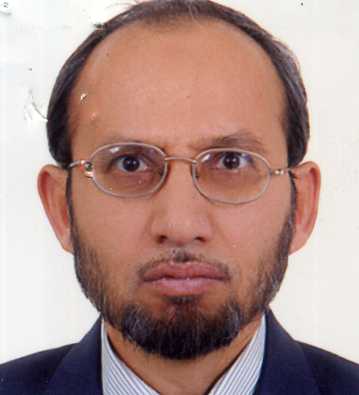
Biography:
Altaf H. Khan has completed three master degrees in Biostatistics (2004), Applied Mathematics (1999) and Mechanical Engineering (2003) from the University of Utah. Currently he is working as a Senior Biostatistician at King Abdullah International Medical Research (National Guard Health Affairs), Riyadh (Saudi Arabia) and prior to that worked at the University of Utah Hospital and Prince Sultan Cardiac Center. He has many publications in international journals and proceedings.
Abstract:
The Fourier analysis, named after the French mathematician Joseph Fourier, is based upon the infinite sum of trigonometric functions such as sine and cosine. Its variant based approaches have wide applicability in almost every branch of sciences, ranging from the study astrophysics to biological sciences. In this work an attempt has been made to discuss briefly the underlying theories pertaining to the Fourier analysis and its vast applicability in statistical sciences will be highlighted, specifically the utilization of the wavelet theory. A wavelet is a waveform of effectively limited duration and has an average of value zero; it is like a short wave which oscillates and has amplitude: it starts at zero, increases/decreases and comes back again to zero; it circumvents the frequency/time issues which occurs in Fourier transform. Fourier transform is a special case of the continuous wavelet transform with the choice of a mother wavelet: e-2πit, where i = √(-1). Wavelets examine a signal or an image in a flexible way while a Fourier transform describes an overall picture of the dataset’s spectrum. Wavelets can easily handle non-stationary objects while the Fourier based approach fails to comprehend such problems. Application of wavelet’s theory in time series analysis, signal processing, dimension reduction, nonparametric regression (shrinkage), density estimation, inverse problem, and compression of noisy signals and images will be outlined along with illustrative examples with real data, will be presented.
Keynote Forum
Altaf H Khan
King Abdullah International Medical Research Center
National Guard Health Affairs
Saudi Arabia
Keynote: Some applications of Fourier /wavelets transform in Statistical Sciences
Time : 10:30-11:00
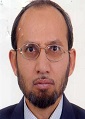
Biography:
Altaf H. Khan has completed three master degrees in Biostatistics (2004), Applied Mathematics (1999) and Mechanical Engineering (2003) from the University of Utah. Currently he is working as a Senior Biostatistician at King Abdullah International Medical Research (National Guard Health Affairs), Riyadh (Saudi Arabia) and prior to that worked at the University of Utah Hospital and Prince Sultan Cardiac Center. He has many publications in international journals and proceedings.http://biometrics-biostatistics.conferenceseries.com/
Abstract:
The Fourier analysis, named after the French mathematician Joseph Fourier, is based upon the infinite sum of trigonometric functions such as sine and cosine. Its variant based approaches have wide applicability in almost every branch of sciences, ranging from the study astrophysics to biological sciences. In this work an attempt has been made to discuss briefly the underlying theories pertaining to the Fourier analysis and its vast applicability in statistical sciences will be highlighted, specifically the utilization of the wavelet theory. A wavelet is a waveform of effectively limited duration and has an average of value zero; it is like a short wave which oscillates and has amplitude: it starts at zero, increases/decreases and comes back again to zero; it circumvents the frequency/time issues which occurs in Fourier transform. Fourier transform is a special case of the continuous wavelet transform with the choice of a mother wavelet: e-2πit, where i = √(-1). Wavelets examine a signal or an image in a flexible way while a Fourier transform describes an overall picture of the dataset’s spectrum. Wavelets can easily handle non-stationary objects while the Fourier based approach fails to comprehend such problems. Application of wavelet’s theory in time series analysis, signal processing, dimension reduction, nonparametric regression (shrinkage), density estimation, inverse problem, and compression of noisy signals and images will be outlined along with illustrative examples with real data, will be presented.
- Track 2: Statistical and Computing Methods; Track 5: Bioinformatics; Track 6: Computer Science and Systems Biology
Location: Texas B

Chair
Francisco Louzada
University of Sao Paulo
Brazil

Co-Chair
Abdel-Salam Gomaa Abdel-Salam
Qatar University
Qatar
Session Introduction
Joel Michalek
University of Texas
USA
Title: p53-based strategy to reduce hematological toxicity of chemotherapy: A pilot study
Time : 11:25-11:45
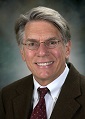
Biography:
Joel E Michalek completed his PhD from Wayne State University. He has a broad background in biostatistics pertaining to theory and methods, preclinical and clinical trials, and epidemiology. He has written protocols and grants, analyzed data, and co-authored manuscripts arising from clinical studies in surgery, emergency medicine, cancer, and pediatrics and was formerly Principal Investigator of the Air Force Health Study, a 20-year prospective epidemiological study of veterans who sprayed Agent Orange and other herbicides in Vietnam. He has authored 180 journal articles and two book chapters.
Abstract:
p53 activation is the primary mechanism underlying pathological responses to DNA-damaging agents such as chemotherapy and radiotherapy. Study objectives were to: (1) define the lowest safe dose of arsenic trioxide that blocks p53 activation in patients and (2) assess the potential of LDA to decrease hematological toxicity from chemotherapy. Patients scheduled to receive a minimum of 4 cycles of myelosuppressive chemotherapy were eligible. For objective 1, dose escalation of LDA started at 0.005 mg/kg/day for 3 days. This dose satisfied objective 1 and was administered before chemotherapy cycles 2, 4 and 6 for objective 2. CBC was compared between the cycles with and without LDA pretreatment. p53 level in peripheral lymphocytes was measured on day 1 of each cycle by ELISA essay. Subjects received arsenic at cycles 2, 4 and 6 and no arsenic at cycles 1, 3, and 5. Of a total of 30 evaluable patients, 26 were treated with 3-week cycle regimens and form the base of our analyses. The mean white blood cell, hemoglobin and absolute neutrophil counts were significantly higher in the suppressed group relative to the activated group. These data support the proof of concept that suppression of p53 could lead to protection of normal tissue and bone marrow in patients receiving chemotherapy.
Hsin-Hsiung Huang
University of Central Florida
USA
Title: The out-of-place testing for genome comparison
Time : 11:45-12:05
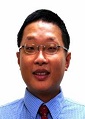
Biography:
Hsin-Hsiung Huang has completed his PhD from the University of Illinois at Chicago and has been a One-Year Visiting Assistant Professor of the Department of Statistics at the University of Central Florida. He is now a Tenure-Track Assistant Professor of the Department of Statistics at the University of Central Florida. He has published 5 papers in reputed journals.
Abstract:
The out-of-place distance measure with alignment-free n-gram based method has been successfully applied to automatic text or natural languages categorization in real time. Like k-mers, n-grams are sub-sequences of length ‘n’ from a given sequence, but the ways of computing the number of n-grams and k-mers are different. Additionally, it is not clear about its performance and the selection of ‘n’ for comparing genome sequences. In this study, the author proposed a symmetric version of the out-of-place measure, a non-parametric out-of-place measure, and an approach for finding the optimal range of ‘n’ to construct a phylogenetic tree with the symmetric out-of-place measures. This approach, then, is applied to four mitochondrial genome sequence data-sets. The resulting phylogenetic trees are similar to the standard biological classification. It shows that the proposed method is a very powerful tool for phylogenetic analysis in terms of both classification accuracy and computation efficiency.
Dongmei Li
University of Rochester
USA
Title: An evaluation of statistical methods for DNA methylation microarray data analysis
Time : 12:05-12:25
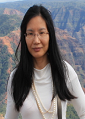
Biography:
Dongmei Li completed her PhD in Biostatistics from Department of Statistics at The Ohio State University. She is currently an interim Associate Professor in the Department of Clinical & Translational Research at the University of Rochester School of Medicine and Dentistry. She has published more than 25 methodology and collaborative papers in reputed journals and been served as Co-Investigator or Statistician on multiple federal funded grants and contracts.
Abstract:
DNA methylation offers a process for elucidating how epigenetic information affects gene expression. β values and M values are commonly used to quantify DNA methylation. Statistical methods applicable to DNA methylation data analysis span a number of approaches such as Wilcoxon rank sum test, t-test, Kolmogorov-Smirnov test, permutation test, empirical Bayes method, and bump hunting method. Selection of an optimal statistical method, however, can be challenging when different methods generate inconsistent results for the same data set. We compared six statistical approaches relevant to DNA methylation microarray analysis in terms of false discovery rate control, statistical power, and stability through simulation studies and a real data example. Our results provide guidance for optimal statistical methods selection under different scenarios. For DNA methylation data analysis purposes, similar results are obtained using either β or M values in terms of false discovery rate control, power, and stability. The empirical Bayes method is recommended for DNA methylation studies with small sample size. All methods are acceptable for medium or large sample sizes. The bump hunting method has much lower stability than the other methods when the true proportion of differentially methylated loci is large, a caveat to be considered when choosing the bump hunting method.
Abdel-Salam Gomaa Abdel-Salam
Qatar University
Qatar
Title: Outlier robust non-linear mixed model estimation on gene expression
Time : 12:25-12:45
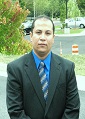
Biography:
Abdel-Salam Gomaa Abdel-Salam holds BS and MS (2004) degrees in Statistics from Cairo University and MS (2006) and PhD (2009) degrees in Statistics from Virginia Polytechnic Institute and State University (Virginia Tech, USA). Prior to joining Qatar University as an Assistant Professor and a Coordinator of the Statistical Consulting Unit, he taught at Faculty of Economics and Political Science (Cairo University), Virginia Tech, and Oklahoma State University. Also, he worked at J P Morgan Chase Co. as Assistant Vice President in Mortgage Banking and Business Banking Risk Management Sectors. He has published several research papers and delivered numerous talks and workshops. He was awarded a couple of the highest prestige awards such as Teaching Excellence from Virginia Tech, Academic Excellence Award, Freud International Award, and Mary G Natrella Scholarship from American Statistical Association (ASA) and American Society for Quality (ASQ), for outstanding Graduate study of the theory and application of Quality Control, Quality Assurance, Quality Improvement, and Total Quality Management. He is a Member of the ASQ and ASA. Also, he was awarded the Start-Up Grant Award from Qatar University (2014/15) and The Cairo University Award for international publication in 2014. His research interests include all aspects of industrial statistics and economic capital models, including statistical process control, multivariate analysis, regression analysis, exploratory and robust data analysis, mixed models, non-parametric and semi-parametric profile monitoring, health-related monitoring and prospective public health surveillance.
Abstract:
In standard analyses of data well-modeled by a Non-Linear Mixed Model (NLMM), an aberrant observation, either within a cluster, or an entire cluster itself, can greatly distort parameter estimates and subsequent standard errors. Consequently, inferences about the parameters are misleading. This paper proposes an outlier robust method based on linearization to estimate fixed effects parameters and variance components in the NLMM. An example is given using the 4-parameter logistic model and gene expression and environment datasets and comparing the robust parameter estimates to the non-robust estimates.
Francisco Louzada
University of Sao Paulo
Brazil
Title: A Unified Multivariate Survival Model in Presence of a Cure Fraction
Time : 12:45-13:05
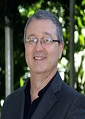
Biography:
Francisco Louzada has completed his PhD from University of Oxford. He is the director of Technology Transfer and Executive Director of External Relations of the Center for Research, Innovation and Dissemination of Mathematical Sciences in Industry (CEPID-CeMEAI), in Brazil. He has published more than 190 papers in reputed journals and has been serving as Editor-in-Chief of the Brazilian Journal of Probability and Statistics (BJPS).
Abstract:
In this talk I present a new lifetime model for multivariate survival data with a surviving fraction. The model is developed under the presence of m types of latent competing risks and proportion of survival individuals. Inference is based on maximum likelihood approach. A simulation study is performed in order to analyze the coverage probabilities of confidence intervals based on the asymptotic distribution of the maximum likelihood estimates. The proposed modeling is illustrated through a real dataset on medical area.
Xing Li
Mayo Clinic
USA
Title: Workshop on Rcircle: A gene-oriented R package for integrating multiple ‘-omics’ data to prioritize disease related hub genes and network
Time : 13:40-14:40
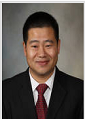
Biography:
Xing Li has completed his PhD in Bioinformatics from The University of Michigan at Ann Arbor. He is an Assistant Professor in Division of Biomedical Statistics and Informatics, Department of Health Science Research at Mayo Clinic which has been recognized as the best hospital for 2014-2015 by US News & World Report. He has published more than 18 papers in reputed journals and has been serving as Peer Reviewers of over a dozen of journals, such as Genomics, BMC Bioinformatics, Stem Cell Research, PLOS ONE, Physiological Genomics, etc.
Abstract:
Biomedical Science has entered the big data era and biologists today have access to an overwhelming abundance of data due to the rapid advancement of high-throughput technology in sequencing and microarray during last decade. The tremendous volume, high dimensions and different types of data pose an unprecedented challenge on data visualization and integration for efficient data exploration and effective scientific communication. Herein, we developed an extendable gene-oriented R package to integrate and visualize interactome (especially for gene interaction networks with large number of nodes), time-course transcriptome data (especially for transcriptional data with more than three time points up to dozens of stages), disease related genetic factors, and disease affected pathways/networks to facilitate the gene prioritization and knowledge discovery. This gene-oriented R package is powerful for visualizing and integrating multiple ‘-omics’ data to prioritize actionable genes and facilitate biomedical knowledge discovery. Package is freely available on R website. One paper with the application of Rcircle package in human Dilated Cardiomyopathy study is featured as cover story on the Journal of Human Molecular Genetics and another paper is recommended as featured article by the Editor of Physiological Genomics. In this workshop, I will demonstrate the usage of this package in prioritizing disease-related genes in Congenital Heart Defects by integrating time-course transcriptome data, interactome data (gene interaction information), disease information, and involved pathway function groups, etc. In addition, the package is flexible to integrate other types of ‘–omics’ data as well, including whole genome sequencing, exome-seq, etc.
Fulvia Pennoni
University of Milano-Bicocca
Italy
Title: Casual analysis of the relation between epigenetic pathways and air pollution based on the joint use of mixed latent Markov models and the propensity score method
Time : 14:40-15:00
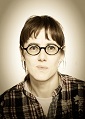
Biography:
Fulvia Pennoni has completed her PhD in Statistics from Florence University and her Postdoctoral studies from Joint Research Centre of the European Commission. After being a Researcher, now, she is an Associate Professor of Statistics at the Department of Statistics and Quantitative Methods of University of Milano-Bicocca. She recently published two books and several articles in the main international statistical journals. She has been serving as Referee in journals on the field of mathematical and statistical models.
Abstract:
We propose a novel model for longitudinal studies based on random effects to capture unobserved heterogeneity. We aim at extending the latent Markov Rasch model, which is specially tailored to deal with confounders and missing data on the primary response. The model is based on both time-fixed and time-varying latent variables having a discrete distribution. In particular, time-varying latent variables are assumed to follow a Markov chain. The model estimation is performed by the maximum likelihood procedure through the EM algorithm. This estimation is based on a set of weights associated to each subject to balance the composition of different sub-samples corresponding to different treatments/exposures in a perspective of causal inference. These weights are computed by the propensity score method. The model is applied to the analysis of epidemiological and molecular data from the Normative Aging Study (NAS), a longitudinal cohort of older individuals to identify key epigenetic pathways in humans that reflect air pollution exposure and predict worse cognitive decline. The participants are assigned estimates of black carbon exposure, a measure of diesel particles, since 2010; have epigenome-wide Illumina Infinium 450K Methylation BeadChip data for methylation at ~486,000 DNA sites measured at two different time points; and are administered cognitive testing assessing multiple functional domains every 3-5 years. We will consider DNA methylation as a possible intermediate variable mediating the effects of air pollution on cognitive aging. Epigenetic profiles may represent cumulative biomarkers of air pollution exposures and aid in the early diagnosis and prevention of air pollution-related diseases.
Ken Williams
KenAnCo Biostatistics
University of Texas
USA
Title: A meta-analysis of meta-analyses comparing LDL cholesterol, non-HDL cholesterol, and apo-lipoprotein B as markers of vascular risk
Time : 15:00-15:20
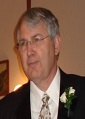
Biography:
Ken Williams received a BS in Applied Math from Georgia Tech in 1971 and an MS in Operations Research from the Air Force Institute of Technology in 1980. He served in the US Air Force for 22 years in Computer Systems and Scientific Analysis. He also served 10 years as a Biostatistician at the University of Texas Health Science Center at San Antonio where he remains an Adjunct Faculty Member. He has been a Freelance Biostatistician with KenAnCo Biostatistics since 2007. Designated a Professional Statistician (PStat) in the inaugural 2011 litter, he has published more than 100 papers in peer-reviewed journals.
Abstract:
This talk will combine and compare two meta-analyses. One included all the published epidemiological studies that contained estimates of the relative risks of LDL-C, non-HDL-C, and apoB predicting fatal or non-fatal ischemic cardiovascular events. Twelve independent reports, including 233,455 subjects and 22,950 events, were analyzed. Standardized relative risk ratios and confidence intervals were LDL-C: 1.25 (1.18, 1.33), non-HDL-C: 1.34 (1.24, 1.44) and apoB: 1.43 (1.35, 1.51), 5.7%>non-HDL-C (p<0.001) and 12.0%>LDL-C (p<0.001). The other meta-analysis included 7 placebo-controlled statin trials in which LDL-C, non-HDL-C, and apoB values were available. Mean CHD risk reduction (95% CI) per standard deviation decrease in each marker across these 7 trials were 20.1% (15.6%, 24.3%) for LDL-C; 20.0% (15.2%, 24.7%) for non-HDL-C; and 24.4% (19.2%, 29.2%) for apoB, 21.6% (12.0%, 31.2%)>LDL-C (p<0.001) and 24.3% (22.4%, 26.2%)>non-HDL-C (p<0.001). The inverse of treatment HRs from the trial meta-analysis were similar to the risk ratios from the observational meta-analysis indicating that parameters from both kinds of studies may be useful for projecting the number of events which can be avoided under different preventive treatment strategies.
Abdelsalam G Abdelsalam
Qatar University
Qatar
Title: Workshop on Advanced statistical analysis using SPSS
Time : 15:20-16:20
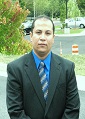
Biography:
Abdelsalam G Abdelsalam holds BS and MS (2004) degrees in Statistics from Cairo University and MS (2006) and PhD (2009) degrees in Statistics from Virginia Polytechnic Institute and State University (Virginia Tech, USA). Prior to joining Qatar University as an Assistant Professor and a Coordinator of the Statistical Consulting Unit, he taught at Faculty of Economics and Political Science (Cairo University), Virginia Tech, and Oklahoma State University. Also, he worked at J P Morgan Chase Co. as Assistant Vice President in Mortgage Banking and Business Banking Risk Management Sectors. He has published several research papers and delivered numerous talks and workshops. He was awarded a couple of the highest prestige awards such as Teaching Excellence from Virginia Tech, Academic Excellence Award, Freud International Award, and Mary G Natrella Scholarship from American Statistical Association (ASA) and American Society for Quality (ASQ), for outstanding Graduate study of the theory and application of Quality Control, Quality Assurance, Quality Improvement, and Total Quality Management. He is a Member of the ASQ and ASA. Also, he was awarded the Start-Up Grant Award from Qatar University (2014/15) and The Cairo University Award for international publication in 2014. His research interests include all aspects of industrial statistics and economic capital models, including statistical process control, multivariate analysis, regression analysis, exploratory and robust data analysis, mixed models, non-parametric and semi-parametric profile monitoring, health-related monitoring and prospective public health surveillance.
Abstract:
This workshop is designed to provide advanced and common statistical techniques in various disciplines which are very important for researches and practitioners using the SPSS. The workshop is designed especially to demonstrate some techniques and applications of biostatistics in medical, public health, epidemiology, pharmaceutical and biomedical fields. The following topics will be dealt with in the workshop: • Introduction to the Inferential Statistics and SPSS, • Perform hypothesis testing and confidence interval analysis for one and two populations, • One and two-way factorial ANOVA with SPSS, • Post Hoc multiple comparisons, • Conducted a Pearson correlation and created a scatter plot for the results, • Perform linear regression analysis (simple and multiple) and logistic regression, and • How to interpret the SPSS outputs and get the results into the report.
Adel Aloraini
Al Baha University
Saudi Arabia
Title: A directed acyclic graphical approach with ensemble feature selection for a better drug development strategy using partial knowledge from KEGG signaling pathways
Time : 16:35-16:55
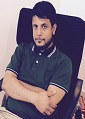
Biography:
Adel Aloraini received Master’s degree from Informatics School at Bradford University, UK in 2007. Then, he received his PhD degree from Computer Science Department at the University of York, UK in 2011. In 2013, he was appointed as the Head of Computer Science Department and later was nominated as the Dean of Computer Science and Information Technology College- Al Baha University. He has been the Head of Machine Learning and Bioinformatics Group at Qassim University since 2012 and recently has been allocated as Associate Member at York Centre for Complex Systems Analysis (YCCSA), York University, UK in 2015. He also has been involved in several program committees worldwide and is being a Reviewer in different journals.
Abstract:
Genes and proteins along KEGG signaling pathways are grouped according to different criteria such as gene duplication and its co-operativity. Gene duplication is a process by which a chromosome or a segment of DNA is duplicated resulting in an additional copy of the gene which undergoes mutations, thereby creating a new different functional gene that shares important characteristics with the original gene. Similar sequences of DNA building blocks (nucleotides) are an example of such duplication. Gene co-operativity is another criterion for grouping genes and proteins together into one family in KEGG signaling pathways that could be manifested at the protein-protein interaction level. At large, KEGG signaling pathways show a high level knowledge of the structural interaction between genes but with some limitations. In this presentation, we will discuss our recent approach for revealing more details about inter-family interactions in KEGG signaling pathways, using gene expression profiles from Affymetrix microarrays which in turn show a promising avenue for a better drug development strategy. Learning from gene expression profiles is, however, problematic given that the number of genes usually exceeds the number of samples, known as the p>>n problem. Learning from such high-dimensional space needs solvers to consider the most relevant genes that best explain the cellular system being studied. Hence, in this presentation we will show how we tackled this problem through developing a machine learning of graphical model approach that utilizes novel ensemble feature selection methods to justify the choice of the most relevant features, genes in this case.
Ajit Kumar Roy
Central Agricultural University Tripura
India
Title: Web resources for bioinformatics, biotechnology and life sciences research
Time : 16:55-17:15
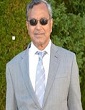
Biography:
Ajit Kumar Roy obtained his MSc degree in Statistics and joined Agricultural Research Service (ARS) of Indian Council of Agricultural Research (ICAR) as a Scientist in 1976. He has edited eighteen books and several conference proceedings covering the areas of statistics, bioinformatics, economics, and ICT applications in aquaculture/fisheries/agriculture and allied fields besides published over 100 articles in refereed journals & conference proceedings. He is a highly acclaimed researcher and consultant. His recent popular books are ‘Applied Big Data Analytics’; ‘Self Learning of Bioinformatics Online’; ‘Applied Bioinformatics, Statistics and Economics in Fisheries Research’ and ‘Applied Computational Biology and Statistics in Biotechnology and Bioinformatics’. He is widely recognized as an expert research scientist, teacher, author, hands-on leader in advanced analytics. He served as National Consultant (Impact Assessment), Consultant (Statistics), Computer Specialist and Principal Scientist at various organizations at National and International levels. Presently he is a visiting Professor of four Indian Universities.
Abstract:
The vast amount of information generated has made computational analysis critical and has increased demand for skilled bioinformaticians. There are thousands of bioinformatics and genomics resources that are free and publicly accessible. However, trying to find the right resource and to learn how to use the complex features and functions can be difficult. In this communication, I explore ways that you can quickly find and effectively learn how to use resources. It will include a tour of examples, resources, organized by categories such as Algorithms and Analysis tools, expression resources, genome browsers, Literature and text mining resources. One can learn how to find resources with the OpenHelix free search interface. OpenHelix searches hundreds of genomics resources, tutorial suites, and other material to deliver the most relevant resources in seconds. Documented the Web-based tools and technologies, resources, web content, blog posts, videos, webinars, and web sites that will facilitate easy access and use that saves time and effort avoiding massive generalized searches or hunting and picking through lists of databases. The purpose of the documentation is to bridge the gap between the rising information needs of biological and medical researchers and the rapidly growing number of online bioinformatics resources. The freely available, searchable databases arranged by categories and sub-categories such as Databases and Analysis Tools, Proteomics Resources, and Enzymes and Pathways. Key programming tools and technologies used in bioinformatics and molecular evolutionary research are provided. Those interested in learning basic biocomputing skills will find links to selected online tutorials.
Nazanin Nooraee
Eindhoven University of Technology
The Netherlands
Title: Strategies for handling missing outcomes in longitudinal questionnaire surveys
Time : 17:15-17:35

Biography:
Nazanin Nooraee has completed her PhD in 2015 from University of Groningen, the Netherlands. Her main interest is in applied statistics with longitudinal analysis orientation. Currently, she is a Postdoctoral Research Fellow at Eindhoven University of Technology, the Netherlands.
Abstract:
Missing data is a pervasive issue in data collection, particularly in questionnaire data. Questionnaire is a tool for obtaining data from individuals, and commonly a (predefined) function of these data (i.e., sum scale or mean scale) is considered to be analyzed. Having, even one unanswered item (question) leads to a missing score. Not tackling this issue may result in biased parameter estimates and misleading conclusions. Although, numerous methods have been developed for dealing with missing data, comparing their performance on questionnaire data has received less attention. In the current study, the performance of different missing data methods were investigated via simulation. We used maximum likelihood and multiple imputation approaches either at item level or at scale level. Furthermore, we implemented a hybrid approach that uses the advantages of both aforementioned methods. Parameter estimates were examined in terms of bias and Mean Square Error (MSE) relative to an analysis of the full data set.
Mohammed Imran
University of Dammam
Saudi Arabia
Title: Bio-stastistics with computational intelligence
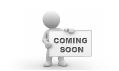
Biography:
Mohammed Imran has completed his PhD from Jamia Millia Islamia (A Central University), New Delhi, India. He is presently working as Assistant Professor in Department of Computer Science, University of Dammam. He has published more than 25 papers in reputed journals and conferences and has been serving as an Editorial Board Member of repute.
Abstract:
In the world of imprecise, partial, and vague information, the crisp logic or is no more found to be producing good results, especially in medical science images. On the other hand, the fuzzy logic defines multiple values of output for different imprecise, partial and vague information in images as input. In such cases, the decision making with crisp logic does not define any fuzzy valid solutions, also called as f-valid solutions. Certainly, in most of the cases fuzzy valid solutions plays an important role, which cannot be neglected. Therefore, the fuzzy valid solution for multiple parameters is calculated using Ordered Weighted Averaging.
Mohammad Imran
King Faisal University
Saudi Arabia
Title: Machine learning approaches for biomedical and biometric data
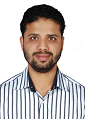
Biography:
Mohammad Imran received PhD in Computer Science in 2012. The title of his thesis was ‘Some Issues Concerning Biometric system’ under the guidance of Professor G Hemantha Kumar. During 2012-13, he had been a Post-Doctorate Fellow under TRC (The Research Council, Oman) sponsored program. Currently, he is working as an Assistance Professor in King Faisal University, Saudi Arabia, Prior to this, he was working as an Associate Consultant at WIPRO Technolgoies, Bangalore. His areas of research interests include machine learning, pattern recognition, computer vision, biometrics, image processing, predictive analysis, algorithms, data structure, linear algebra. He authored 25 international publications which include journals and peer-reviewed conferences.
Abstract:
The aim of this talk is to apply a particular category of machine learning and pattern recognition algorithms, namely the supervised and unsupervised methods, to both biomedical and biometric images/data. This presentation specifically focuses on supervised learning methods. Both methodological and practical aspects are described in this presentation. The presentation is in two parts. In the first part, I will introduce data preparation concepts involved in preprocessing. After a quick overview, I will give an overview dimensions /features, curse of dimensionality, understanding data impurities like missing data and outliers, data transformations, scaling, estimation, normalization, smoothening, etc. In the second part of the presentation, I will discuss issues and challenges specific to 1. Supervised and unsupervised learning, 2. Statistical learning theory, 3. Errors and noise, 4. Bias and variance tradeoff, 5. Theory of generalization, 6. Training vs. testing, and 7. Over-fitting vs. under-fitting. A whole summarization of learning concept and its applications will be done. During the course of the presentation, I will attempt to survey some results on biometric and biomedical data. Finally, future challenges will be discussed.
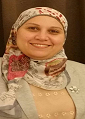
Biography:
Amal Khalifa is currently working as an Assistant Professor of Computer Science at College of Computer & Information Sciences, Princess Norah University in KSA. In addition, she is the Vice-Head of the Computer Science Department and a Member in the accreditation committee of the program. She graduated in 2000 from the Scientific Computing Department at Faculty of Computers & Information Science, Ain Shams University, Egypt. She worked as a Teaching Assistant and earned her MSc in the field of Information Hiding in Images in 2005. In 2006, she was granted a scholarship in a joint supervision program between the Egyptian Ministry of Higher Education and University of Connecticut-USA to get the PhD in the area of high performance computing in 2009. Her main research interests are: steganography and watermarking applications, high performance computing, cloud computing, computational biology and security.
Abstract:
People are always looking for secure methods to protect valuable information against unauthorized access or use. That's why disciplines like cryptography and steganography are gaining a great interest among researchers. Although the origin of steganography goes back to the ancient Greeks, recent Information Hiding techniques embed data into various digital media such as sound, images, and videos. However, in this research we took a step further to utilize DNA sequences as a carrier of secret information. Two techniques will be presented. The first method hides the secret message into some reference DNA sequence using a generic substitution technique that is followed by a self-embedding phase such that the extraction process can be done blindly using only the secret key. The second technique is called the Least Significant Base Substitution or LSBase for short. This method exploits a remarkable property of codon redundancy to introduce silent mutations into DNA sequences in such a way that the carrier DNA sequence can be altered without affecting neither the type nor the structure of protein it produces.
- Young Researchers Forum
Session Introduction
Juliana Torres Sánchez
Universidad Nacional de Colombia
Colombia
Title: Effect of atypical populations in meta-analysis
Time : 17:35-17:45
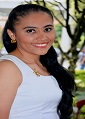
Biography:
Juliana Torres Sánchez has completed her Undergraduation at Universidad Nacional de Colombia, and she is now studying for her Graduate program in Statistics at the same university in Colombia. She has published an article called ‘Efecto de niveles crecientes de nitrógeno no protéico dietario sobre la concentración de precursores gluconeogénicos en hígado bovino’ in the Journal Facultad Nacional De Agronomía; Medellín ISSN: 0304-2847 ed: Universidad Nacional de Colombia v.63 fasc.N/A p.5363 - 5372, 2010.
Abstract:
The meta-analysis is a statistical technique that allows the sum of different studies, to obtain conclusions allowing the unification of results, helping for true understanding of the response variable analyzed. In this research, the effects that an atypical study has on the results of the meta-analysis are discussed, and provide recommendations for dealing with them, where the interest response, refers to mean and/or proportions. General Objectives: To generate a proposal about the identification and the appropriate handling of atypical studies in meta-analysis. Specific Objectives: To determine the effect of an atypical study over meta-analysis results, to introduce the methodology by using simulation in ‘metafor’ package from R software and developing the corresponding conclusions and suggest solutions to detect and deal with atypical studies. Methods or Models: This research project is based on the realization of a meta-analytic process to evaluate the effect of atypical populations in the process of meta-analysis through simulations using statistical software that supports control to assess variations in the mean and variance of only one study that is considered atypical, under control levels. The simulation procedure is based on shifts in mean and variance both up and down, so that their effects can be observed in the meta-analysis to infer the effect in other types of study of various features, without diminishing the validity. The incidence that atypical study takes in an analytical process may be negligible or can be so significant that it can radically change the meaning or own inferences reached by the meta-analysis, offering expressions that could be taken as true and false or vice versa.
Rachel Oluwakemi Ajayi
University of Kwazulu-Natal
South Africa
Title: The association of under nutrition and cognitive outcomes among 4-6 years old South African children
Time : 17:45-17:55
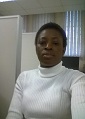
Biography:
Rachel Oluwakemi Ajayi is a PhD student in Statistics, University of KwaZulu-Natal with research in the area of identifying factors which influence the Cognitive Development of Children in impoverished communities. She had a Master of Science in Statistics from University of Lagos, Nigeria, with research on Error Analysis of Generalized Negative Binomial and Bachelor of Science in Statistics from University of Ilorin, Nigeria, with research on Statistical Quality Study, Characterization and Control of Faults reported to NITEL. She has published one paper in reputed journal and is currently working on a manuscript.
Abstract:
Background: The study investigated 4-6 years old children’s health, nutritional status and cognitive development in a predominantly rural area of Kwazulu-Natal, South Africa. Methods: This was the baseline of a longitudinal cohort study (Asenze) Phase 1 study of pre-school children in a rural area of Kwazulu-Natal, South Africa. The study investigated the association of demographic variables, site (geographic area), child’s HIV status, child’s haemoglobin level, anthropometric measures (height-for-age z-scores), weight-for-age z-scores, mid-upper arm circumference on children’s cognitive performance measured by Grover counter and Kauffman’s KABC-11 subtests. The use of General Linear Models was employed to determine the effect of the predictors while the study also incorporated factor analysis to create global cognitive scores. Result: Based on the data, the effect of haemoglobin, sex and weight-for-age were not as significant as other factors. The principal factors of children’s cognitive outcomes were site, education, height-for-age, mid-upper arm-circumference, HIV status and age. Children who had any low cognitive scores came from poorer sites, had less pre-school education, and were older; while HIV positive children most likely had low scores in height-for-age and mid-upper-arm circumference. Conclusion: There is a need to improve the nutrition of children in this region of Kwazulu-Natal, in order to improve their cognitive outcomes.
Rowena F Bastero
University of Maryland
USA
Title: A swapping method based on covariate classification for average treatment effect estimation
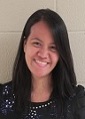
Biography:
Rowena F Bastero has completed her Master’s degree in the University of the Philippines and is currently completing her PhD in the University of Maryland, Baltimore County. At present, her areas of interest are propensity score analysis and meta-analysis under the guidance of Dr Bimal K Sinha. Her other area of research is spatio-temporal modeling, where she has published a paper entitled “Robust Estimation of a Spatiotemporal Model with Structural Change” in the Journal of Communications and Statistics – Simulation and Computation.
Abstract:
In observational studies, systematic differences in the covariates of the treatment and control groups may exist which pose a problem in estimating the average treatment effect. Although propensity score analysis provides a remedy to this issue, assessment made on the matched pairs or groups formed through these scores continue to reflect imbalance in the covariates between the two groups. Hence, a modified method is proposed that guarantees a more balanced group with respect to some, if not all, possible covariates and consequently provide more stable estimates. This matching procedure estimates the average treatment effect using techniques that infuse “swapping” of models based on classical regression and meta-analyses procedures. The “swapping” procedure allows for the imputation of the missing potential outcome Y(1) and Y(0) for units in the control and treatment groups, respectively while meta-analysis provides a means of combining the effect sizes calculated from each matched group. Simulated and real data sets are analyzed to evaluate comparability of estimates derived from this method and those formulated based on propensity score analysis. Results indicate superiority of the estimates calculated from the proposed model given its smaller standard errors and high power of the test. The proposed procedure ensures perfect balance within matched groups with respect to categorical variables and addresses issues of homogeneous effect sizes. It also identifies and incorporates relevant covariate information into the estimation procedure which consequently allows derivation of less biased estimates for the average treatment effect.
Som B Bohora
The University of Oklahoma Health Sciences Center
USA
Title: Generalization of the semi-parametric AUC regression model with discrete covariates
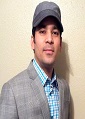
Biography:
Som B Bohora is working as Research Biostatistician at the Behavioral and Developmental Pediatrics section at OUHSC and is also a Student at the College of Public Health. He was trained in biostatistics and epidemiology, and has research experience in Fetal Alcohol Spectrum Disorders (FASD), HIV/AIDS clinical trials and child maltreatment prevention. He is interested in the applications of statistical computing, predictive analytics, and dynamic reporting in these research arenas.
Abstract:
In this research, we considered data with a non-normally distributed response variable. In particular, we extended an existing AUC model that handles only two discrete covariates to a generalized AUC model that can be used on data with any number of discrete covariates. Comparing with other similar methods which requires iterative algorithms and bootstrap procedure, our method involved only closed-form formulae for parameter estimation, hypothesis testing, and confidence intervals. The issue of model identifiability was also discussed. Our model has broad applicability in clinical trials due to the ease of interpretation on model parameters and its utility was illustrated using data from a clinical trial study aimed at evaluating education materials for prevention of Fetal Alcohol Spectrum Disorders (FASDs). Finally, for a variety of design scenarios, our method produced parameters with small biases and confidence intervals with nominal coverages as demonstrated by simulations.
Dereje W Gudicha
Tilburg University
The Netherlands
Title: Power Analysis for the Likelihood Ratio Test in Latent Markov Models: Short-cutting the bootstrap p-value based method
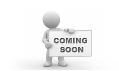
Biography:
Gudicha is a prospective Ph.D student at Tilburg University, The Netherlands. His PhD dissertation, supervised under Professor Jeroen K. Vermunt, deals with power and sample size computation methods for both simple mixture models for cross-sectional data and complex mixture models for longitudinal data. The results of this dissertation contribute to the field of mixture modelling in many ways: address factors that affect the power of statistical tests for mixture distributions, but also, considering the case of hypothesis testing for which the asymptotic theory is not warranted, present a method for power/sample size computation. Gudicha got his master in applied statistics from Addis Ababa University and research master in social and Behavioral science from Tilburg University. He has several years of teaching at the University and has also published his research work on high quality journals.
Abstract:
In recent years, the latent Markov (LM) model has proven useful to identify distinct unobserved states and transitions between these states over time in longitudinally observed responses. The bootstrap likelihood ratio (BLR) test is becoming a gold standard for testing the number of states, yet little is known about power analysis methods for this test. This paper presents a short-cut to a p-value based power computation for the BLR test. The p-value based power computation involves computing the power as the proportion of the bootstrap p-value (PBP) for which the null hypothesis is rejected. This requires to perform the full bootstrap for multiple samples of the model under the alternative hypothesis. Power computation using the short-cut method involves the following simple steps: obtain the parameter estimates of the model under the null hypothesis, construct the empirical distributions of the likelihood ratio under the null and alternative hypotheses via Monte Carlo simulations, and use these empirical distributions to compute the power. The advantage of this short-cut method is that it is computationally cheaper and is simple to apply for sample size determination.